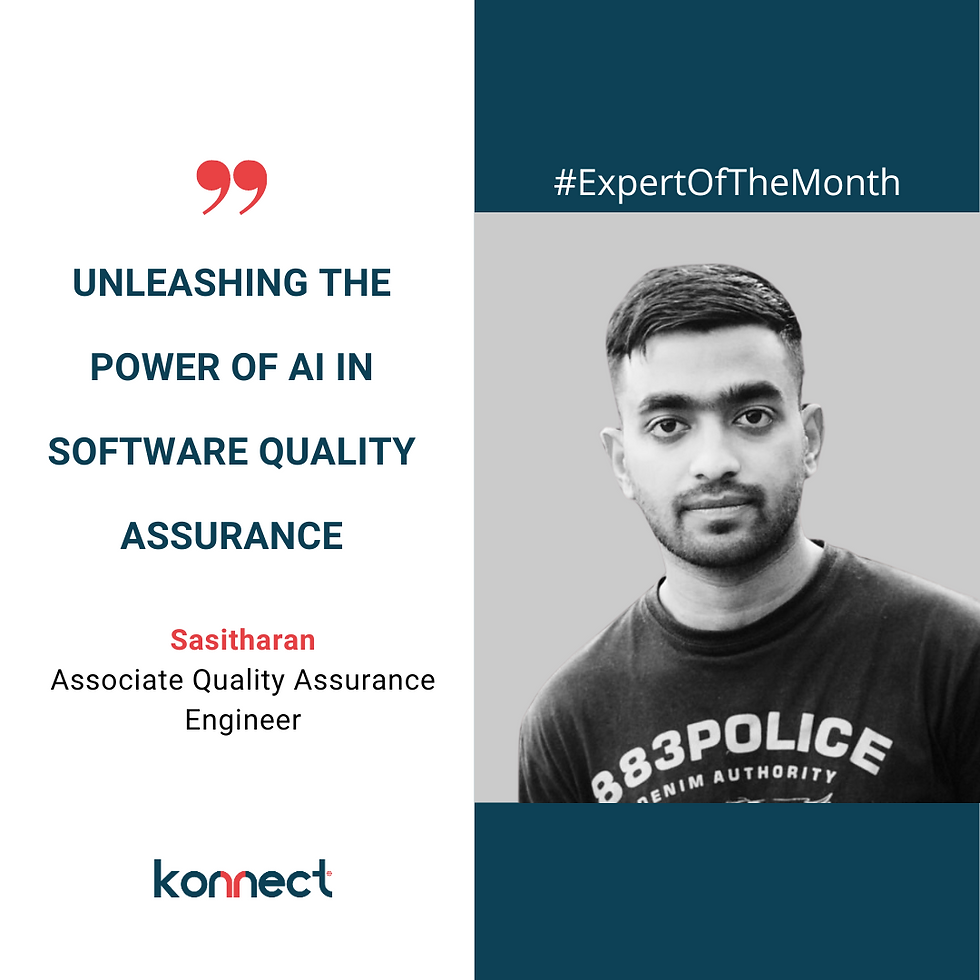
In recent years, the field of software quality assurance has witnessed a transformative wave with the integration of Artificial Intelligence (AI) and Machine Learning (ML) technologies. As an Associate QA Engineer, I've had the opportunity to explore and implement these cutting-edge solutions, and I believe it's a game-changer for the entire industry. The software development life cycle is dynamic, and testing methodologies are no exception. In this blog post, I will discuss the impact of AI in software quality assurance, shedding light on its benefits, challenges, and the potential future it holds for our field.
Improved Test Automation:
AI-driven test automation tools have significantly enhanced efficiency by intelligently identifying repetitive test cases and automating them. This has not only reduced the testing time but also increased test coverage and overall product quality.
Predictive Analysis for Defect Prevention:
Utilizing ML algorithms for predictive analysis allows us to identify potential defects and vulnerabilities in the early stages of development. This proactive approach has helped our team in preventing issues before they escalate, saving time and resources.
Enhanced Test Case Design:
AI assists in the generation of intelligent and data-driven test cases, ensuring that the most critical aspects of the application are thoroughly tested. This has led to more robust test scenarios and improved test suite effectiveness.
Dynamic Test Data Generation:
AI-driven algorithms can dynamically generate test data, covering a wide range of scenarios. This not only ensures comprehensive testing but also helps in uncovering potential issues related to data integrity, security, and performance.
Adaptive Test Maintenance:
Traditional test scripts require constant maintenance due to changes in the application. AI-powered testing tools can adapt to changes autonomously, reducing the manual effort required for script updates. This adaptability is particularly valuable in agile development environments.
Behavioral Driven Development (BDD) with AI:
Incorporating AI into BDD practices enhances collaboration between development and QA teams. Natural Language Processing (NLP) algorithms can analyze and understand user stories, facilitating the creation of more accurate and relevant test cases.
Real-time Performance Monitoring:
AI-based performance testing tools offer real-time monitoring and analysis of application performance under various conditions. This enables us to identify and address performance bottlenecks before they impact end-users.
AI for Regression Testing:
Regression testing, often a time-consuming process, is streamlined with AI. Machine learning algorithms can intelligently select and prioritize test cases based on code changes, reducing the regression testing cycle and accelerating the release process.
Challenges and Ethical Considerations:
Acknowledging the potential challenges and ethical considerations is crucial. As we embrace AI in QA, it's essential to address issues such as bias in AI models, data privacy concerns, and the need for continuous monitoring to ensure the reliability of AI-driven testing .
Training and Up skilling:
The integration of AI in QA requires a paradigm shift in skills. It's essential for QA professionals to engage in continuous learning and up skilling to effectively leverage these technologies. Training programs and resources should be made available to ensure the team is well-equipped for this technological shift.
In conclusion, the infusion of AI in Software Quality Assurance is reshaping the way we approach testing. While challenges exist, the benefits are substantial, leading to more efficient, accurate, and proactive testing processes. As an Associate QA Engineer, embracing and mastering these AI-driven tools positions us at the forefront of innovation in the software testing landscape. I hope these additional points contribute to a more comprehensive and engaging blog post. Please feel free to provide feedback or suggest any specific areas you'd like me to focus on further.
Best regards,
Sasitharan
コメント